RoadSavior – Revolutionizing Crisis Response with Satellite Imagery and Machine Learning
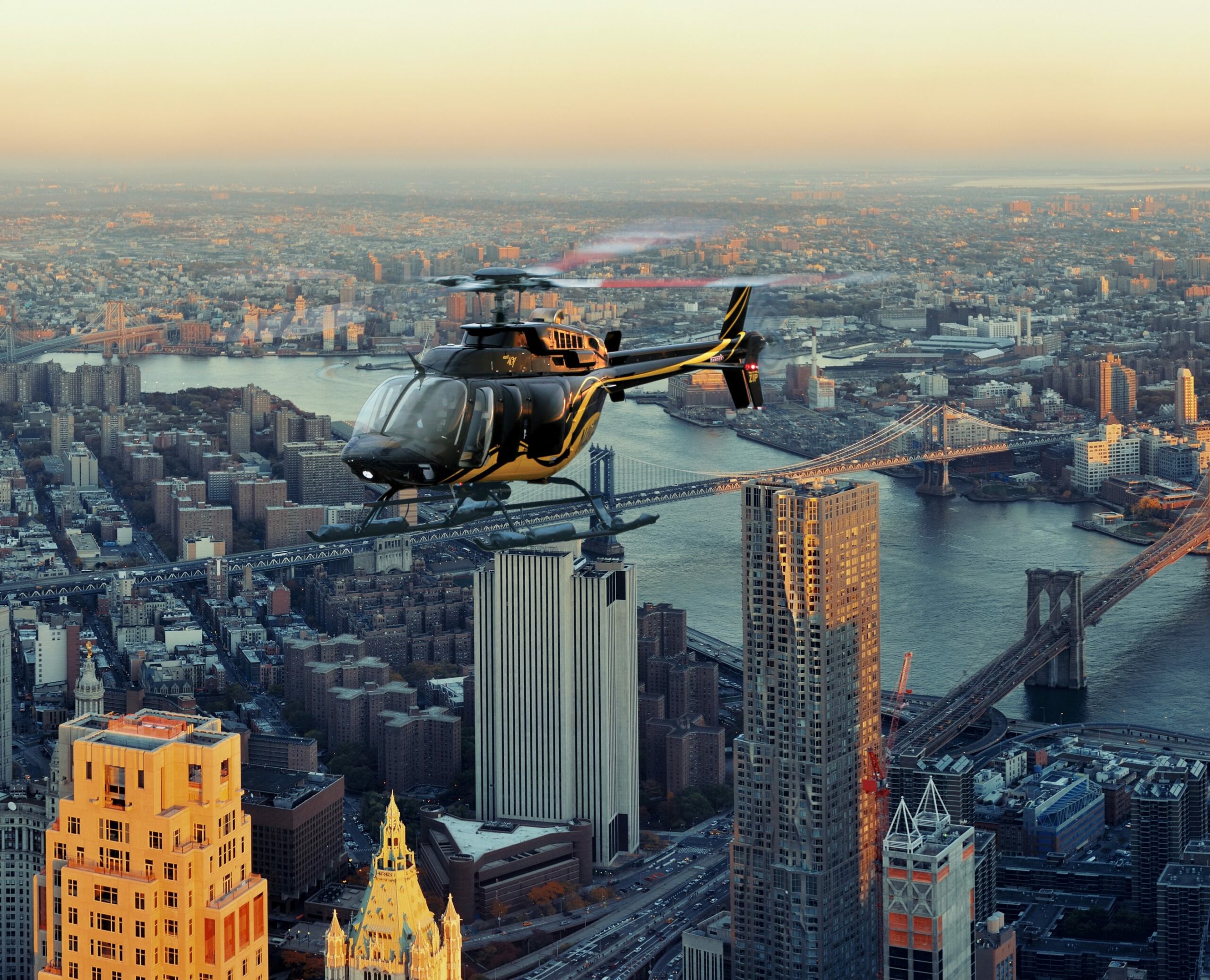
Unleashing the Power of Machine Learning for a Safer Tomorrow
We live in a world where disasters can strike without warning, leaving communities shattered and countless lives at risk. In these dire moments, every second counts. That’s where RoadSavior comes. It is a machine learning model that segments roads from aerial images with stunning accuracy. By doing this, it paves the way for faster and more effective crisis response. This culminated as a result of me desiring real world projects which I am passionate about, to place on my resume for potential employers. I was tired of the same old datasets used in bootcamps. I wanted to show where my passions and energy lie in life. That’s when the machine learning for crisis response project came to life.
The Ultimate Dataset: DeepGlobe Road Extraction Challenge
To build a hypothetical life-saving machine learning model, I needed the best dataset available. The DeepGlobe Road Extraction Challenge provided me with high-resolution aerial images and road masks that served as the foundation for my model. This incredible dataset accelerated the progress and allowed me to focus on developing a world-class solution.
Mastering Data Preprocessing: The Key to AI Success
In the quest to create a machine learning model that could change lives, I knew that preprocessing was vital. I split the dataset into training and validation subsets, ensuring the model would be tested against a wide range of scenarios. With a custom DataGenerator class, I loaded and preprocessed images and masks in batches. This optimized them for the ML model’s learning process.
The U-Net: A Cutting-Edge ML Architecture That Saves Lives
To tackle the complex task of road segmentation, I turned to the U-Net. U-Net is an advanced convolutional neural network known for its prowess in segmentation tasks. This powerful architecture allowed me to create a model that could quickly and accurately detect roads in aerial images, even in the most challenging conditions.
Training RoadSavior: The ML Model That Learns to Save Lives
I embarked on a thrilling journey to train the hypothetical life-saving machine learning model, fine-tuning hyperparameters and employing advanced optimization techniques. With each epoch, RoadSavior grew smarter and more accurate, unlocking its potential to revolutionize crisis response efforts.
RoadSavior in Action: A New Era of Crisis Response
After tireless research, development, and training, RoadSavior achieved a remarkable 97.56% training accuracy and 97.09% validation accuracy. These groundbreaking results show that machine learning can make a real difference in search and rescue missions, ultimately saving lives and improving disaster response.
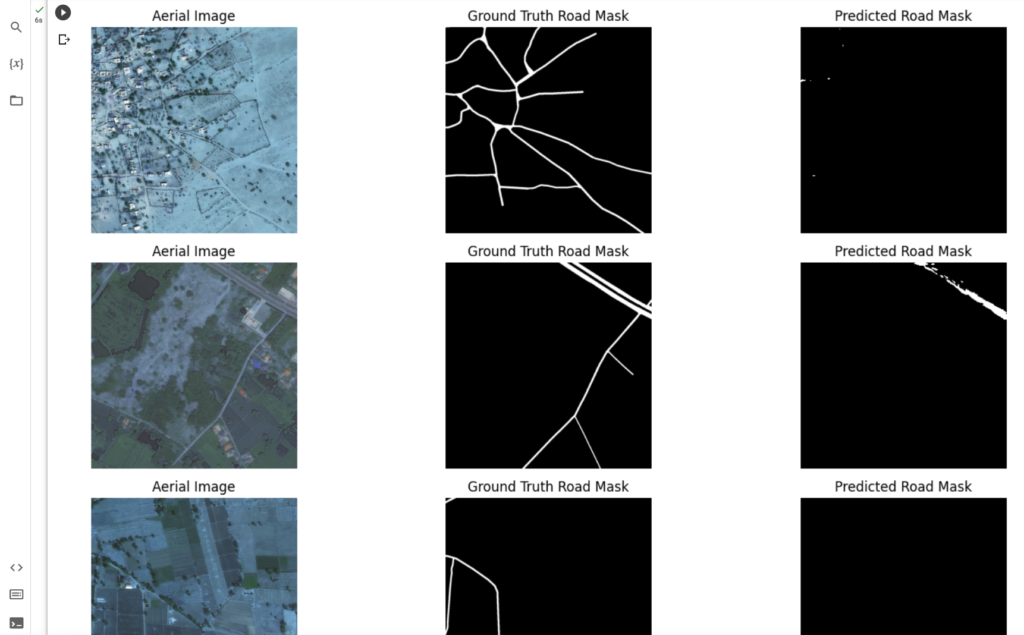
Acknowledging the Heroes Behind the Scenes
None of this would have been possible without the dedicated researchers behind the winning results in the DeepGlobe Road Extraction Challenge. Their tireless work in curating and annotating this invaluable dataset laid the foundation for this potential life-saving machine learning model.
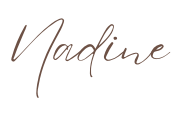